Speak your "customers language" with smart AI agents
AI breaks down communication barriers, ensuring every customer understands how your product solves their problems.
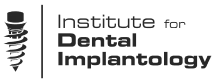
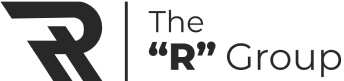
Human-centric optimization process.
Our optimization approach aligns with how your product solves their problems and use AI to breaks down communication barriers
Use Cases:
Case Study A: Entity De-duplication for Enhanced Data Accuracy
Client Profile
Company A - Data Management Platform Provider
Problem Statement:
Company A's platform ingests company data from multiple sources to provide accurate data to clients. However, the company faces significant challenges in understanding the relationships between company entities, necessitating human review.
For example, there may be entities that share the same EIN (Employer Identification Number) but have different names and addresses. By improving entity resolution, Company A aims to enhance the reliability and accuracy of its system.
Mighty Minds Solution
We will support Company A's entity matching efforts using two primary approaches:
LLM-based Entity Deduplication Pipeline
Construct a pipeline through the Mighty Minds platform that deduplicates entities remaining uncertain after Company A's initial deduplication algorithm.
Construct a pipeline through the Mighty Minds platform that deduplicates entities remaining uncertain after Company A's initial deduplication algorithm.
Construct a pipeline through the Mighty Minds platform that deduplicates entities remaining uncertain after Company A's initial deduplication algorithm.
Construct a pipeline through the Mighty Minds platform that deduplicates entities remaining uncertain after Company A's initial deduplication algorithm.
Construct a pipeline through the Mighty Minds platform that deduplicates entities remaining uncertain after Company A's initial deduplication algorithm.
Knowledge Graph Construction
Construct an entity knowledge graph (KG) using VectorShift's internal knowledge graph generation algorithms.
Create nodes in this graph corresponding to entities, storing relevant information for each entity on each node.
Establish edges corresponding to relations between entities.
When new entities are added to the graph, automatically perform the necessary deduplication steps, enriching the graph with any new relevant data.
Enable Company A to query any entity/relation in the graph through a similarity search or a direct graph query.
Allow Company A to edit the graph directly, encoding institutional knowledge into the graph's structure.
Expected Outcomes
- Significantly reduced need for human review in entity resolution.
- Improved accuracy and reliability of Company A's data management system.
- Enhanced ability to identify and merge duplicate entities across various data sources.
- A queryable knowledge graph that continually improves with new data additions.
Case Study B: Automated Internal Reporting for Management
Client Profile
Company B - Large Corporation with Extensive Reporting Needs
Problem Statement:
Company B has an internal reporting team that produces hundreds of reports every year for the leadership team. Each report is an extensive document containing information either
1) pulled directly from various data systems or
2) from various other documents (e.g., historical reports, other written reports). This process is time-consuming and prone to human error.
Mighty Minds Solution
We will support Company B's entity matching efforts using two primary approaches:
Report Generation Tool
Create a tool that allows for the submission of a similar report along with other relevant instructions (e.g., update these numbers with the most up-to-date numbers).
The tool will produce a "first pass" of the report based on the input and instructions.
Intelligent Report Completion
Develop a toolchain that "learns" the relevant sections that go into the report of interest.
Recursively produce additional LLM queries to fill out / complete each section.
For each section, leverage the appropriate data sources and perform necessary data transformations (e.g., for section 1, we need to leverage data source A and B, and perform X data transformation to fit the data in the right way).
Give the model the considerations that employees use today for each "tool" or data source so that the toolchain effectively replicates the employees creating the reports.
Record and present data used in generating the response for auditing purposes.
Expected Outcomes
- Significant reduction in time spent on report generation.
- Increased consistency and accuracy in reports.
- Ability to produce more frequent updates with less human intervention.
- Freeing up of human resources for more strategic tasks.
Case Study C: Intelligent Specs and Contracts Database Search
Client Profile
Company C - Engineering and Construction Firm
Problem Statement:
For example, there may be entities that share the same EIN (Employer Identification Number) but have different names and addresses. By improving entity resolution, Company A aims to enhance the reliability and accuracy of its system.
Mighty Minds Solution
We will build a search engine using the VectorShift platform that can answer niche questions using the company's data as context, along with presenting to the user the most relevant documents that answer the user's question.
Specs Search
Develop a search engine to help with putting together specifications.
Identify similar specification documents (at the subtask level) that have been written for similar projects (based on project executive summary).
Return links and descriptions to the 5 most relevant documents (if available), sorted by recency.
Contract Search
Create a search function to effectively manage tasks / sub-projects under an umbrella contract or conduct relevant reporting (external and internal).
First identify all documents associated with a parent contract number to answer specific questions about the project.
Enable querying for specific contract details such as:
1) Contract date
2) Modification dates
3) Contract ceiling
4) Details on provisions
5) Contracting officers
Expected Outcomes
- Significant time savings in spec writing and contract management.
- Improved accuracy and consistency in spec and contract creation.
- Enhanced ability to leverage existing knowledge and past work.
- Faster response times to client queries about contracts and subcontracts.
Case Study D: Internal Employee Co-pilot for Product Expertise
Client Profile
Company D - Technology Company with Complex Product Portfolio
Problem Statement:
Since its founding, Company D has accumulated a wealth of published research and expertise developed internally on its products. However, mastering this information for any single person is difficult because:
(1) the information is distributed across multiple sources / departments,
(2) the sheer amount of research, and
(3) expertise is often "stored" in the minds of key experts within Company D.
Not completely leveraging the knowledge base can lead to unhappy customers and potential reputational risk. On the other hand, experts / executives are often stretched thin responding to all relevant requests and can't be "everywhere all the time".
Mighty Minds Solution
We will build a solution using the VectorShift platform that involves an interface where the user can perform queries / follow up queries using natural language about research and info on Company D's products.
AI-Powered Co-pilot
Develop an interface for natural language queries about Company D's products and research.
Embed the thinking of Company D experts, such as the prioritization of research by use case.
Create a system that can "magnify" the reach of internal experts by answering / re-directing front line employees to the best resource as if the expert was answering each of the questions individually.
Intelligent Knowledge Base
Aggregate and index all relevant product information, research, and expert knowledge.
Implement advanced semantic search capabilities to retrieve the most relevant information for each query.
Continuously update the knowledge base with new research and product information.
Personalized Responses
Tailor responses based on the user's role and level of expertise.
Provide both high-level summaries and detailed technical information as needed.
Expected Outcomes
- Improved access to company expertise for all employees.
- Reduced burden on key experts for routine inquiries.
- Enhanced customer satisfaction through more informed and timely responses.
- Accelerated onboarding and continuous learning for employees.
- Mitigation of reputational risks associated with inconsistent or incomplete product information..
Selected Work
AI-driven Chatbot for Overseas Educational Consultancy

Driving results through advanced AI technologies
When design met satisfaction and success.
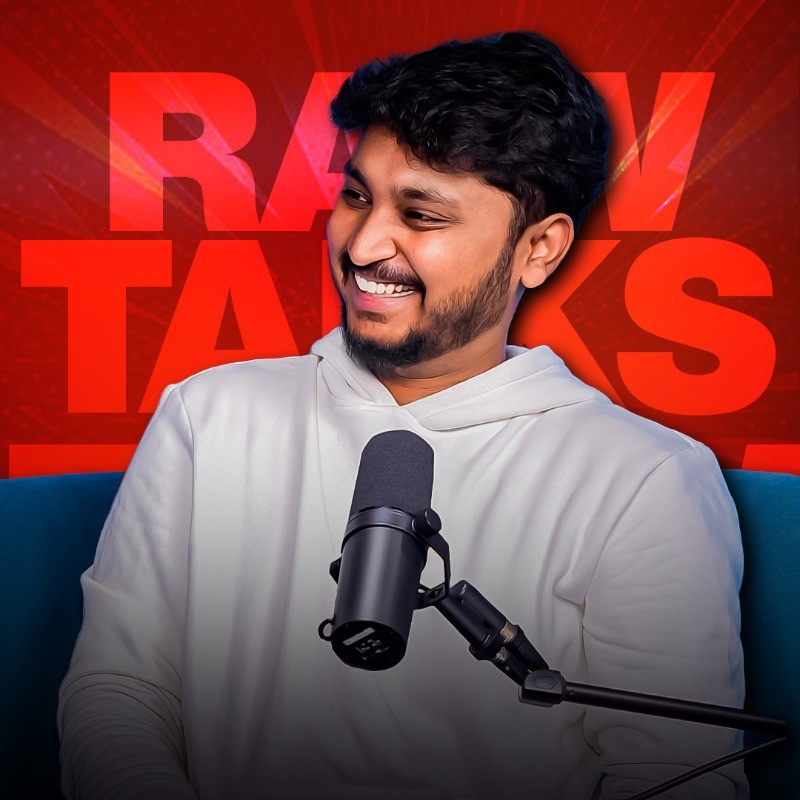

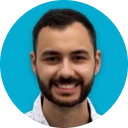
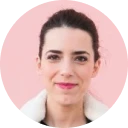
Lorem ipsum dolor sit amet, consectetur adipiscing elit. Suspendisse varius enim in eros elementum tristique. Duis cursus, mi quis viverra ornare, eros dolor interdum nulla, ut commodo diam libero vitae erat. Aenean faucibus nibh et justo cursus id rutrum lorem imperdiet. Nunc ut sem vitae risus tristique posuere.
Frequently asked
There are many variations of passages of Lorem Ipsum available, but the majority have suffered in some form, by injected humour
What is an AI agent?
AI agents are software programs that use artificial intelligence to perform tasks autonomously, such as answering customer queries, providing recommendations, or predicting equipment failures. They can learn from interactions and improve their performance over time.
How do AI agents improve customer service?
AI agents enhance customer service by providing instant responses to inquiries, automating routine tasks, and offering personalized experiences. They can handle multiple interactions simultaneously, reducing wait times and improving overall customer satisfaction.
What metrics can be tracked using AI agents?
AI agents can track various metrics, including goal completion rates, fallback rates, user sentiment, conversion rates, and maintenance efficiency. These metrics help businesses evaluate the performance of their AI systems and optimize their strategies.
How does predictive maintenance work with AI agents?
Predictive maintenance involves using AI agents to analyze data from historical performance to predict when maintenance should be performed. This approach helps prevent unexpected failures and reduces downtime.
Can AI agents operate without human intervention?
Yes, AI agents can operate autonomously to a certain extent, handling routine tasks and making decisions based on programmed algorithms and learned data. However, human oversight is often necessary for complex or nuanced situations.
How do AI agents learn and improve over time?
AI agents learn through machine learning algorithms that analyze data from past interactions. They adapt their responses and actions based on feedback and new information, continually improving their performance and accuracy.
What challenges do businesses face when implementing AI agents?
Challenges include data privacy concerns, integration with existing systems, the need for quality data, and potential resistance from employees. Businesses must address these issues to successfully implement AI agents and maximize their benefits.
The mighty blogs
Discover actionable tips, practical advice, and industry insights that add tangible value to your brand

How to create SVG-ready icon symbols in Sketch
Lorem ipsum dolor sit amet, consectetur adipiscing elit. Suspendisse varius enim in eros elementum tristique. Duis cursus, mi quis viverra ornare, eros dolor interdum nulla, ut commodo diam libero vitae erat. Aenean faucibus nibh et justo cursus id rutrum lorem imperdiet. Nunc ut sem vitae risus tristique posuere.

Cinema 4D Visual Explorations by David Milan
Lorem ipsum dolor sit amet, consectetur adipiscing elit. Suspendisse varius enim in eros elementum tristique. Duis cursus, mi quis viverra ornare, eros dolor interdum nulla, ut commodo diam libero vitae erat. Aenean faucibus nibh et justo cursus id rutrum lorem imperdiet. Nunc ut sem vitae risus tristique posuere.
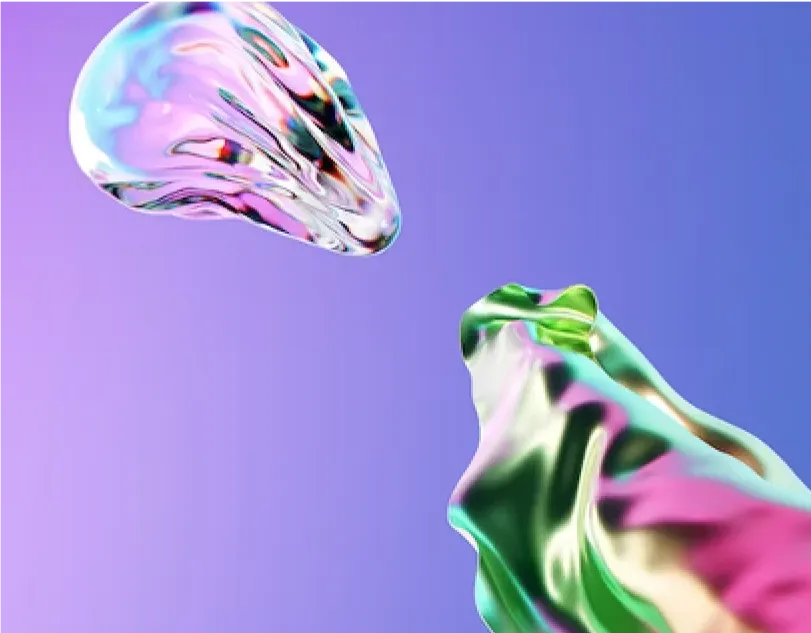
Mental models for designers
Lorem ipsum dolor sit amet, consectetur adipiscing elit. Suspendisse varius enim in eros elementum tristique. Duis cursus, mi quis viverra ornare, eros dolor interdum nulla, ut commodo diam libero vitae erat. Aenean faucibus nibh et justo cursus id rutrum lorem imperdiet. Nunc ut sem vitae risus tristique posuere.
Chat with the chief
Gain transparent clarity through a one-on-one interaction with the founder of Mighty Minds.
